Master post
Subhro Das
Research Staff Member
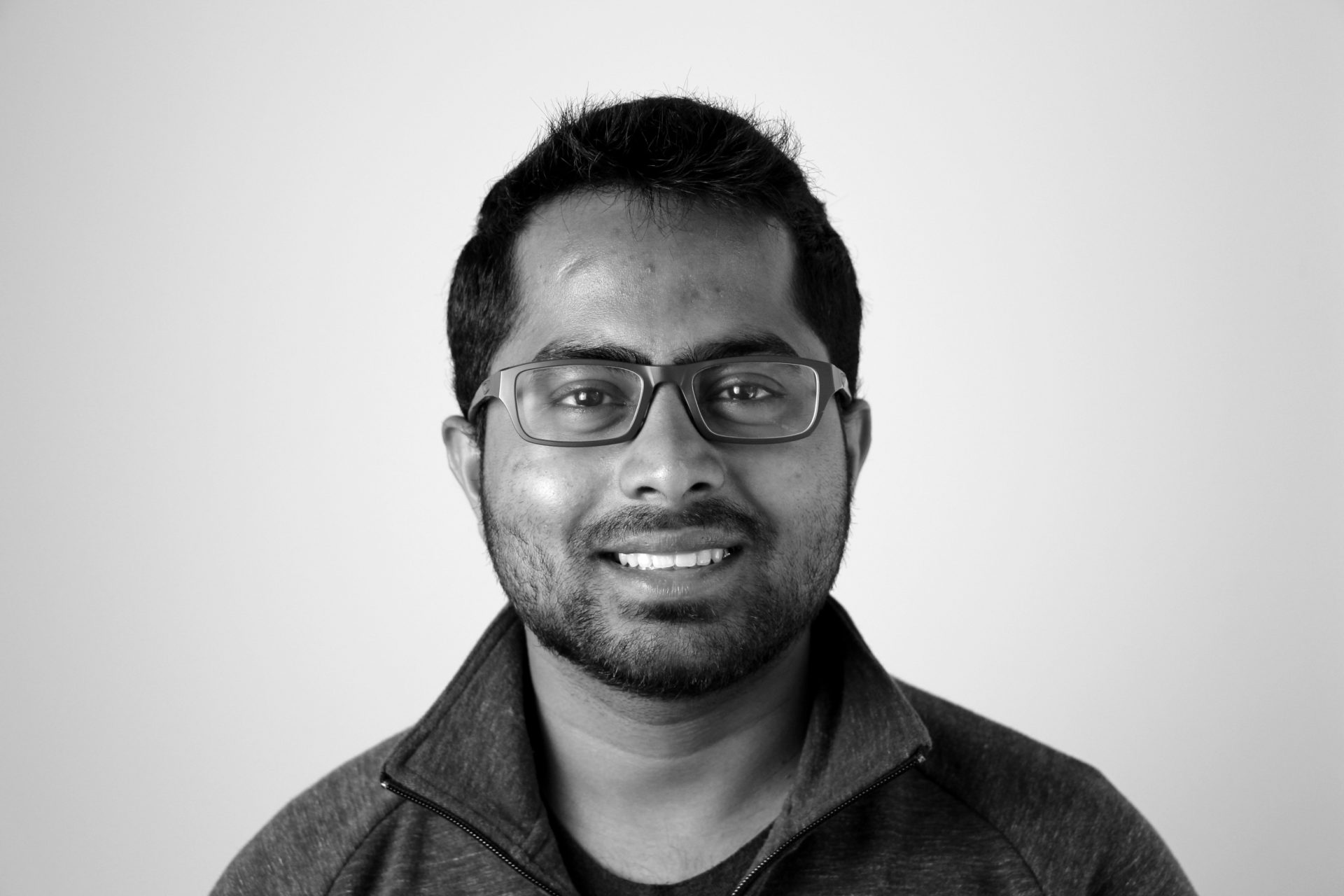
Categories
Subhro Das is a Research Staff Member in AI Algorithms at the MIT-IBM Watson AI Lab, IBM Research, Cambridge MA. He is a Research Affiliate at MIT, co-leading IBM’s engagement in the Bridge pillar of MIT Quest for Intelligence. He serve as the Co-Chair of the AI Learning Professional Interest Community (PIC) at IBM Research.
His research interests are in distributed learning over multi-agent networks, dynamical systems, multi-agent reinforcement learning, accelerated & adaptive optimization methods, and online learning in non-stationary environments – broadly in the areas of AI, machine learning, and statistical signal processing with applications in healthcare and social good. Before moving to Cambridge, he was a Research Scientist at IBM T.J. Watson Research Center, New York. Therein, he worked on developing signal processing and machine learning based predictive algorithms for a broad variety of biomedical and healthcare applications.
He received MS and PhD degrees in Electrical and Computer Engineering from Carnegie Mellon University in 2014 and 2016, respectively. His dissertation research was in distributed filtering and prediction of time-varying random fields and he was advised by Prof. José M. F. Moura. He completed his Bachelors (B.Tech.) degree in Electronics & Electrical Communication Engineering from Indian Institute of Technology Kharagpur in 2011. During the summers of 2009, 2010 and 2015, he was an intern at Ulm University (Germany), Gwangju Institute of Science & Technology (South Korea), and, Bosch Research (Palo Alto, CA), respectively.
Publications with the MIT-IBM Watson AI Lab