Moments in Time Dataset: one million videos for event understanding
Dan Gutfreund
Principal Research Scientist and Senior Manager, AI Models Science
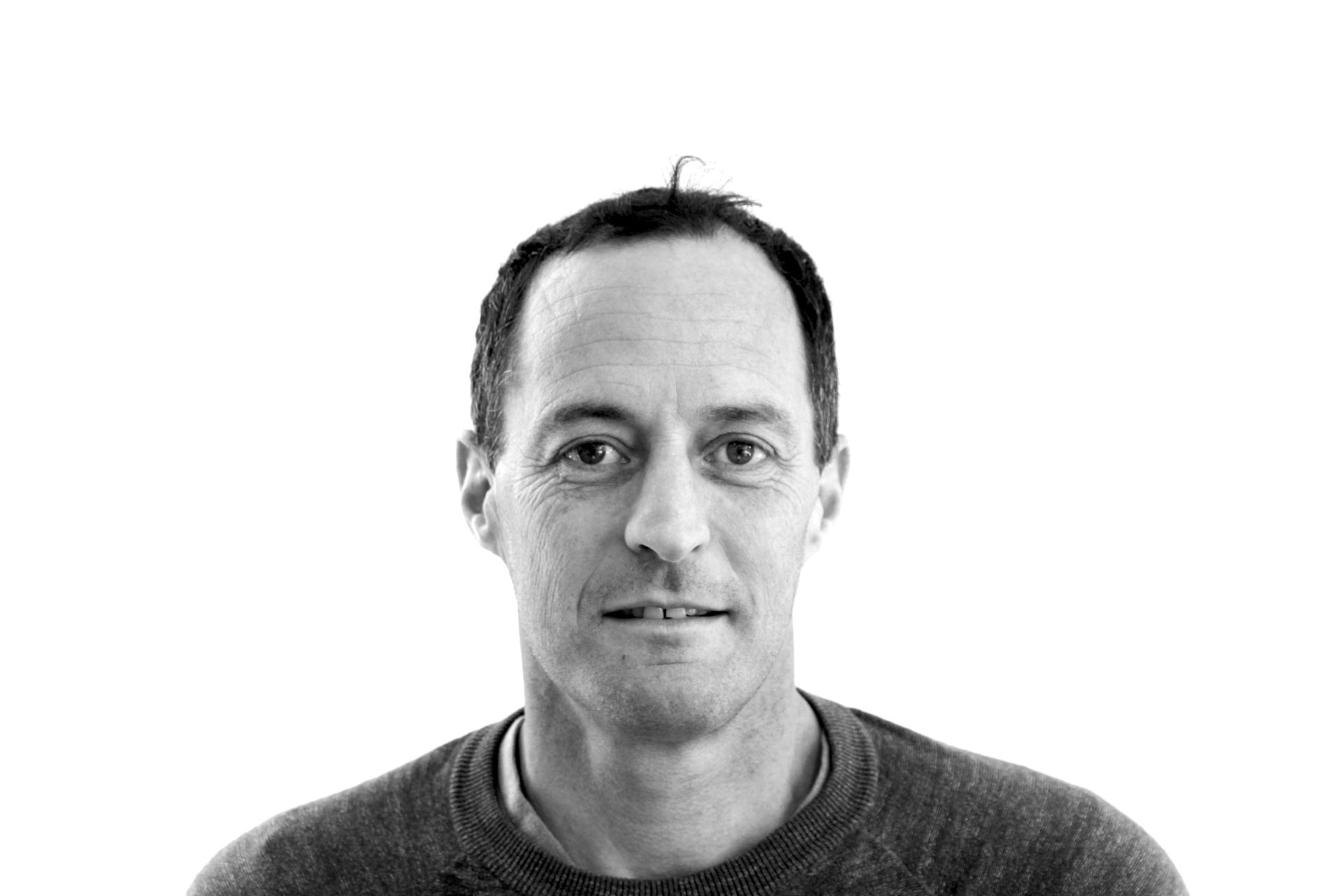
Dan Gutfreund is a principal investigator at the MIT-IBM Watson AI Lab and a manager of the AI Models Science department. Before he moved to the Cambridge research lab, Gutfreund was at the Haifa Research Lab, where he held several managerial and technical leadership positions. In his last role, Gutfreund was the manager in charge of IBM Project Debater. In 2005, he received a PhD in computer science from the Hebrew University in Jerusalem, Israel. Before joining IBM in 2009, he was a postdoctoral fellow and a lecturer at Harvard University and MIT.
Gutfreund’s research interests are in machine learning with applications to natural language processing and computer vision. Previously, he worked on problems in theoretical computer science in the areas of computational complexity and foundations of cryptography.
Top Work

ObjectNet: A bias-controlled dataset object recognition
Publications with the MIT-IBM Watson AI Lab