Tess Smidt
Associate Professor, Department of Electrical Engineering and Computer Science
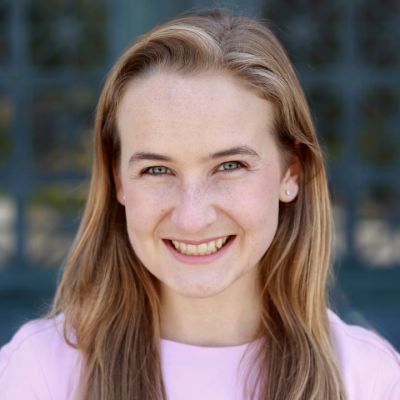
Tess Smidt is an associate professor in the Department of Electrical Engineering and Computer Science (EECS) and principal investigator of the Atomic Architects group at the Research Laboratory of Electronics (RLE) at MIT. Her research focuses on machine learning that incorporates physical and geometric constraints, with applications to materials design. Her research areas of interest include developing neural networks from first-principles for rich data types (e.g. scientific data and geometry), chemical and material science and design, group theory and symmetry, density functional theory, scientific computing, computational geometry, computer-aided design, and rapid prototyping. Prior to joining the MIT EECS faculty, she was the 2018 Luis W. Alvarez Postdoctoral Fellow at Lawrence Berkeley National Laboratory and interned on the Google Accelerated Sciences team, where she developed Euclidean symmetry equivariant neural networks which naturally handle 3D geometry and geometric tensor data. Tess earned her PhD and MA in physics from the University of California, Berkeley; she received her SB in physics from MIT.
Publications
- Batatia, I., Geiger, M., Munoz, J., Smidt, T., Silberman, L., & Ortner, C. (2023). A General Framework for Equivariant Neural Networks on Reductive Lie Groups. 37th Conference on Neural Information Processing Systems (NeurIPS).
- Rackers, J., Tecot, L., Geiger, M., & Smidt, T. (2023). A recipe for cracking the quantum scaling limit with machine learned electron densities. Machine Learning: Science and Technology, 4(1), 015027. https://doi.org/10.1088/2632-2153/acb314
- Merker, H. A., Heiberger, H., Nguyen, L. T. H., Liu, T., Chen, Z., Andrejevic, N., Drucker, N. C., Okabe, R., Kim, S. E., Wang, Y., Smidt, T., & Li, M. (2022). Machine learning magnetism classifiers from atomic coordinates. iScience, 25(10), 105192.
Media
- November 28, 2022: MIT News, The task of magnetic classification suddenly looks easier