Boston-area undergraduates develop a taste for careers in tech at Break Through Tech AI
Authors
Authors
Boston-area undergraduates develop a taste for careers in tech at Break Through Tech AI
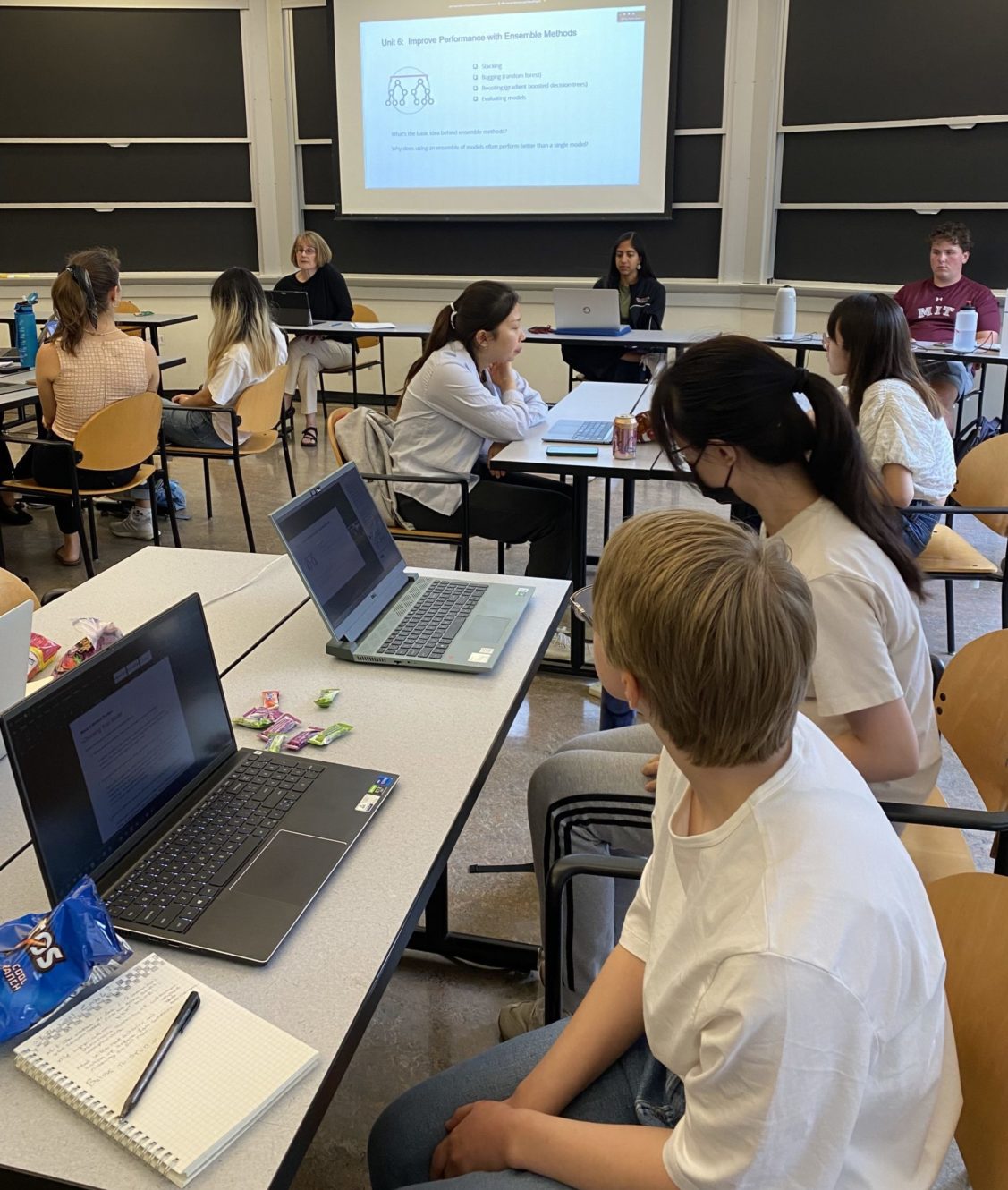
IBM researchers and managers shared how they achieved success in the AI sector during a panel discussion.
There is no shortage of advice for how to break into the tech industry; most crucially, it requires building a strong foundation in technical skills, a community for learning and mentorship, and a portfolio demonstrating experience within a particular niche. Break Through Tech AI, a program launched this summer in the MIT Stephen A. Schwarzman College of Computing in collaboration with Break Through Tech, aims to provide these opportunities for local, women and underrepresented groups graduating with degrees in computing.
“There is a strong appetite within the MIT community to help increase the diversity and competitiveness of those prospecting computing-focused careers, and facilitating more equitable access to opportunities through the Institute’s resources and connections is one of many steps we’re taking to help bring that to fruition,” says Aude Oliva, director of strategic industry engagement and MIT director of the MIT-IBM Watson AI Lab (Lab). “This new avenue bridges academia with industry to provide technical skills with practical insights and mentorship for the benefit of students.”
To support this pipeline of talent development — within fields like data science, machine learning, and artificial intelligence — and diversity and inclusion in industry, the program arranges for AI professionals to share their career experiences — presenting an aspect of their research and their roles within their organizations. On July 26, three IBM researchers and managers joined over 30 Greater Boston-area undergraduate students, who are enrolled in the program from more than 15 institutions and universities, for a virtual, moderated panel discussion, which was followed by skills-based coursework on ensemble methods.
AI strategy manager at IBM and the Lab Austin Little, AI software engineer at the Lab Damilola Adesina, and IBM Research principal research staff member Yada Zhu shared how they use industry-relevant tools to develop real-world solutions, some nuances and exciting challenges of current projects, and interpersonal and business skills they cultivated to build relationships with project partners and companies.
With over 10 years of experience at IBM, Zhu jumped right into her machine learning research “motivated by real-world problems spread [across] the different industries, like energy, manufacturing, ecommerce,” said Zhu. “In the last five years, I have been focusing on AI for finance.” Discussing the digital shift and future of financial services, Zhu shared three pillars of her work: financial crimes compliance, natural language processing and machine learning for financial forecasting and banking, and customer intelligence for loans, risk management, and banking. She handles data, such as sentiment on market movement and environment, time-series information, financial documents and corporate filings, and information with many features, like social media and news reports, that come from and are used by analysts, managers, advisors, and traders. She emphasized that her area of work has the ability to impact, not only individuals, but millions of people, so the results need to be robust, understandable, and fair. “The financial market is in financial services domain, so it’s a highly regulated industry. So, every decision either [has to] be transparent and explainable, so that the regulators and also investors, they could interpret the results to prevent like financial fraud,” said Zhu. She points out that the data and tasks required for this work are heterogeneous, making forecasting challenging. For example, “we are leveraging like both the dynamic market signal, as well as all kinds of textured information, such as financial news, to dynamically optimize their trading decisions. So, here we are developing the reinforcement learning-based model, but we’re using financial news to augment the market stage, and it achieves a very good result here.”
Austin Little focused on his various roles and walked the students through how he connects AI research, with Zhu’s team for example, to companies for real-world impact. Little evolved his undergraduate background in physics with some coding and AI work, into a client-facing consultancy in the life sciences and managerial roles with IBM and the Lab, crafting business cases. Little was strategic in his early years, spending time to build out a professional network and conduct research at a nearby university for two years, picking up machine learning techniques, in addition to some self-taught programming. “A lot of it was seeking out experiences that were outside of the classroom that were able to, kind of, supplement the knowledge that I wasn’t getting from my physics degree,” said Little. “And so that’s how I’ve, kind of, started to bridge the gap between the physics and AI piece … In terms of the business skills, similarly, I sought out other extracurricular programs that helped me build those soft skills. So, I got involved in leadership positions on campus within organizations.”
He transitioned this work into product development and consultancy, working in teams to construct proof-of-concept models, as well as deployment-ready ones for clients, particularly in health care. Little shared that this included using natural language processing to register drug complaints and outcomes. Summing up his career pathway: “…all the research that I did, even if it wasn’t machine learning-based, it was still pretty useful for when I transitioned into the field,” said Little. “If you just understand math really, really well, and you know how to code up some of these algorithms, you can get into some pretty niche roles and some pretty niche spaces that are that are pretty interesting…”
Damilola Adesina’s work threads the needle between Zhu’s and Little’s, prototyping AI systems using synthetic data generators. “Basically, what we do is to, let me say, bring AI to light.” Working within a team, Adesina occupies the B2B, or business to business, space, building models to solve client-driven problems that could then be put into production or applied to various use cases. Adesina shared, while he didn’t pursue a computer science degree, he still specialized in wireless telecommunications, for example device identification and data gathering — fields that are highly regulated and have privacy concerns. “…Because of these, it is important to build synthetic data that can be used to develop models or systems that can be used in our everyday life,” he noted, such as financial systems that have sensitive, personal information.
With synthetic data, Adesina explained, it looks and feels like real data, complies with international laws, reduces risk and cost, and increases the speed and agility of applying it to new models. In their work, Adesina’s team generates new datasets and evaluates them with various methods, like statistical, privacy, detection, and efficacy metrics. He clarifies that they’re not interested in specific values in the data, but the distribution. Adesina helped students to understand, for instance, how inherent biases might be reflected in the generated, synthetic data or how to build datasets with cases of rare incidents.
Drawing on their curriculum training and experiences, the students engaged with each of the guests, querying how the IBM researchers tackled problems or sought opportunities to cultivate skills and/or support.
Following their online coursework over the summer, the students will come to campus during the Fall semester to tackle machine-learning projects in teams. These industry-focused projects will help prepare students for careers in a competitive field, as well as contribute to the students’ work portfolios.
“The students are naturally sparked with curiosity for AI and machine learning, which is evident in their participation in labs, as well as for what a career might look like for them,” says Chu Huang, program director for Break Through Tech AI. “Engagement with real-world professionals is the first step on this road to helping them flesh this out — developing aspirations, confidence and knowledge to thrive in this sector, in addition to exploring the realms of what it could offer them.”