Causal inference is expensive. Here’s an algorithm for fixing that.
Kristjan Greenewald
Research Scientist
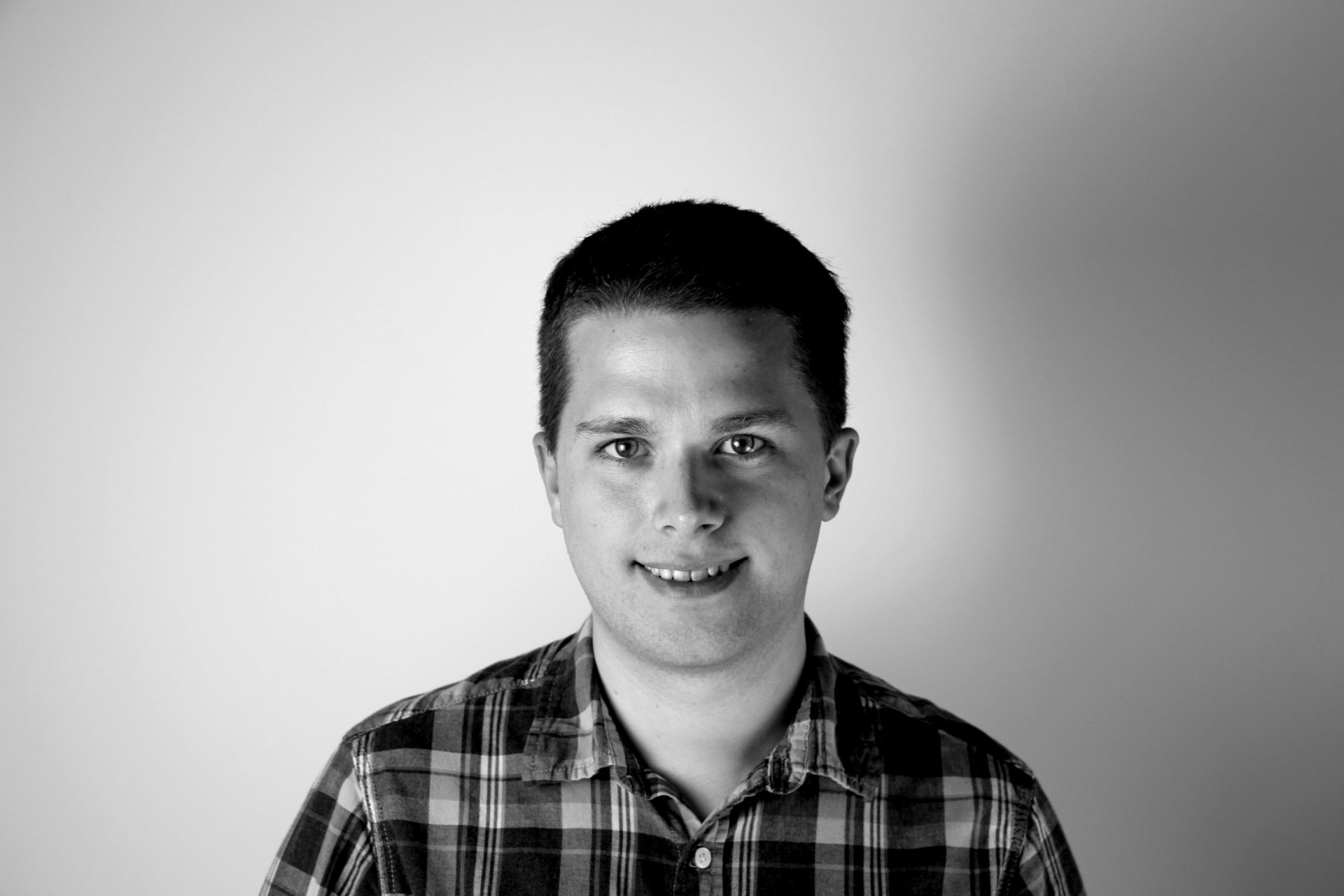
Who they work with
Categories
Kristjan Greenewald is a research scientist at the MIT-IBM Watson AI Lab, serving as principal investigator on multiple funded MIT-IBM research projects. Greenewald received his PhD from the University of Michigan in 2017 focusing on signal processing and machine learning and was a postdoctoral research fellow at the Harvard University Statistics department before joining IBM Research in 2018. His research interests include optimal transport, causal inference, and statistical learning theory, with recent applications including LLM evaluation and alignment, uncertainty quantification, differential privacy, and agentic frameworks.
Publications with the MIT-IBM Watson AI Lab