The Neuro-Symbolic Concept Learner: Interpreting Scenes, Words, and Sentences From Natural Supervision
Joshua Tenenbaum
Co-Scientific Director, MIT Quest for Intelligence; Professor, Brain and Cognitive Sciences; MacArthur Fellow
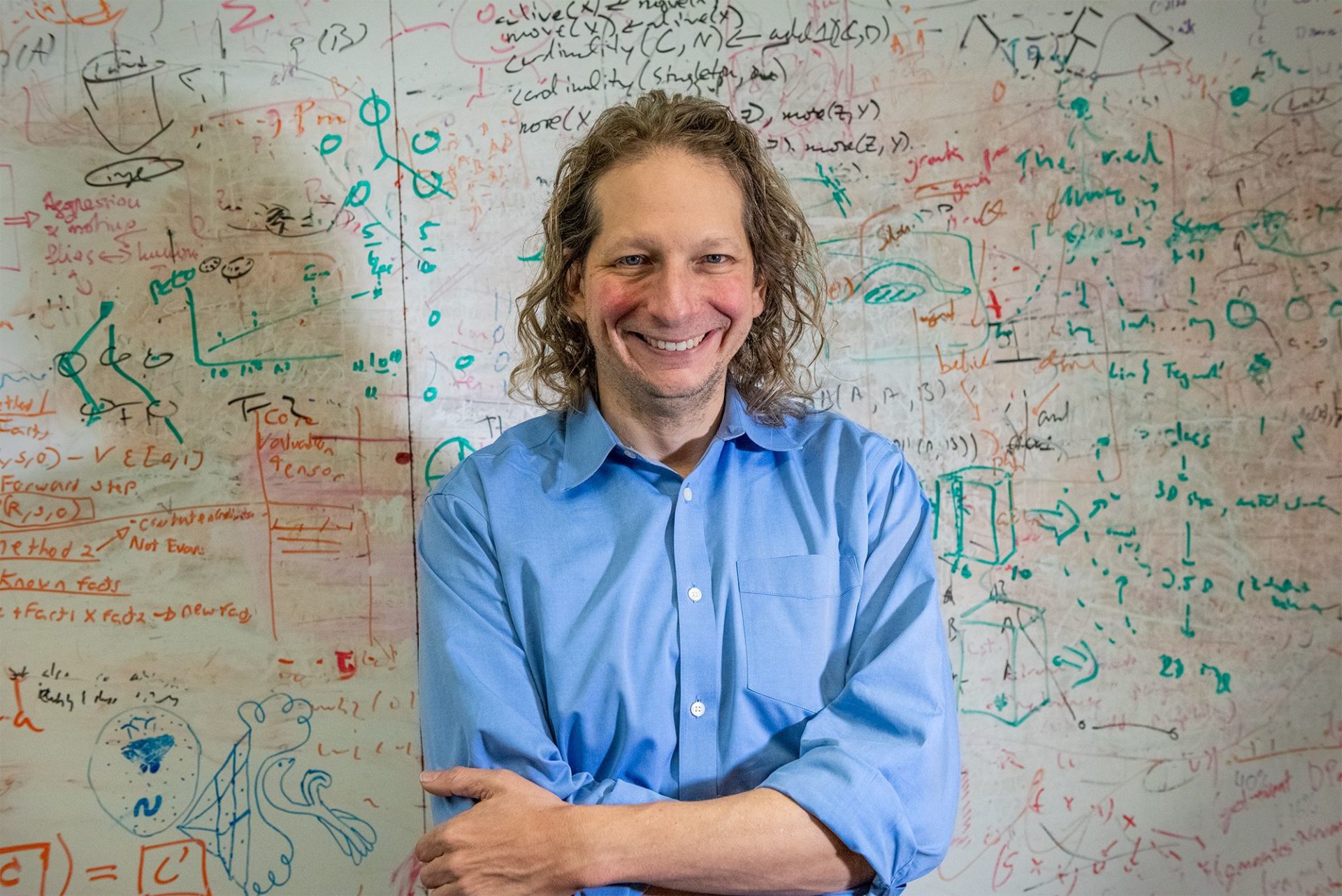
Who they work with
Joshua Tenenbaum is a professor of computational cognitive science in MIT’s Department of Brain and Cognitive Sciences and a co-scientific director with the MIT Quest for Intelligence. He is also an investigator at the Center for Brains, Minds and Machines and the Computer Science and Artificial Intelligence Laboratory. Tenenbaum’s research straddles cognitive science and artificial intelligence, where his goals are to reverse engineer human intelligence and to build machines that behave in human-like ways and have greater use to society. Through a combination of mathematical modeling, computer simulation, and behavioral experiments, Tenenbaum tries to uncover the logic behind our everyday inductive leaps: constructing perceptual representations, separating “style” and “content” in perception, learning concepts and words, judging similarity or representativeness, inferring causal connections, noticing coincidences and predicting the future. Tenenbaum is a MacArthur Fellow and has received the National Academy of Sciences’ Troland Research Award. He earned a BA from Yale University, and a PhD in brain and cognitive sciences from MIT.
Selected Publications
- Ajay, A., Han, S., Du, Y., Li, S., Gupta, A., Jaakkola, T., Tenenbaum, J., Kaelbling, L., Srivastava, A., Agrawal, P. (2023) Compositional Foundation Models for Hierarchical Planning. Conference on Neural Information Processing Systems (NeurIPS).
- Tung, H., Ding, M., Chen, Z., Bear, D.M., Gan, C., Tenenbaum, J., Yamins, D., Fan, J., Smith, K. (2023). Physion++: Evaluating Physical Scene Understanding that Requires Online Inference of Different Physical Properties. Conference on Neural Information Processing Systems (NeurIPS) Datasets and Benchmarks Track.
- Wang, T. J., Zheng, J., Ma, P., Du, Y., Kim, B., Spielberg, A., Tenenbaum, J., Gan, C., Rus, D. (2023). DiffuseBot: Breeding Soft Robots With Physics-Augmented Generative Diffusion Models. Conference on Neural Information Processing Systems (NeurIPS).
- Katz, B., Gutfreund, D., Tenenbaum, J., Shu, T., Kuo, Y-L., Barbu, A., Tejwani, R., Stankovits, B. (2023). Zero-shot linear combinations of grounded social interactions with Linear Social MDPs. Association for the Advancement of Artificial Intelligence (AAAI).
- Gan, C., Li, S., Huang, Z., Chen, T.,Du, T., Su, H., Tenenbaum, J. (2023). DexDeform: Dexterous Deformable Object Manipulation with Human Demonstrations and Differentiable Physics. International Conference on Learning Representations (ICLR).
- Ding, M., Shen, Y., Fan, L., Chen, Z., Chen, Z., Luo, P., Tenenbaum, J., Gan, C. (2023). Visual Dependency Transformers: Dependency Tree Emerges from Reversed Attention. Conference on Computer Vision and Pattern Recognition (CVPR).
- Chitnis, R., Lozano-Perez, T., Silver, T., Tenenbaum, J., Kaelbling, L. (2022). Learning Neuro-Symbolic Relational Transition Models for Bilevel Planning. International Conference on Intelligent Robots and Systems (IROS).
- Gan, C., Zhang, S., Tenenbaum, J., Xu, M., Shen, Y., Lu, Y., Zhao, D. (2022). Prompting Decision Transformer for Few-shot Policy Generalization. International Conference on Machine Learning (ICML).
- Han, J., Huang, W., Ma, H., Li, J., Tenenbaum, J., Gan, C. (2022). Learning Physical Dynamics with Subequivariant Graph Neural Networks. Conference on Neural Information Processing Systems (NeurIPS).
Media
- March 9, 2020: Wired, If AI’s so smart, why can’t it grasp cause and effect?
- Dec. 2, 2019: MIT News, Helping machines perceive some laws of physics.
- Sept. 25, 2019: MIT News, Josh Tenenbaum receives 2019 MacArthur Fellowship.
-
June 14, 2019: MIT News, Toward artificial intelligence that learns to write code.
-
April 2, 2019: MIT News, Teaching machines to reason about what they see.
Publications with the MIT-IBM Watson AI Lab